The wake detection particularly interesting because of the challenges of identifying vessels that turn off their identification systems. Traditionally, optical and radar imaging have been used to locate ships, but these methods encounter difficulties when dealing with small ships or structures that can be confused with natural objects such as icebergs.
The main challenges in ship wake detection center on confusion with other linear structures in the marine environment and the lack of quality dataThe scarcity of labeled datasets limits the training of advanced deep learning models, reducing the accuracy and robustness of detections. In addition, in radar (SAR) and optical imagery, wake features can easily be confused with other natural formations, such as ocean waves, spills or floating debris, complicating accurate identification.
Deep learning-based algorithms have been developed that use “two-step” detection approaches, which first identify possible wake regions and then refine them, and “one-step” approaches, which are faster and more efficient by detecting and classifying wakes directly. These detections can be especially useful for maritime traffic control and homeland defenseoperations; wakes, which can extend several kilometers, provide a detectable trail despite the lack of direct ship signals.
Model input and output
The model for input detection uses VHR (Very High Resolution) and HR optical imaging to detect the Kelvin wakethe main component of the wakes and corresponding to the characteristic “V” shape, together with the turbulent wakewhich forms the line generated by ship propellers. For this purpose, a “one-step” approach based on convolutional neural networksCNNis employed, which demonstrates favorable behavior even on images of lower resolution than those used in their training.
The model output provides in SEDA lines on the Kelvin wake (in green) next to the turbulent wake (in red) converging on the position of the originating ship, as can be seen in Figure 1 and 2.
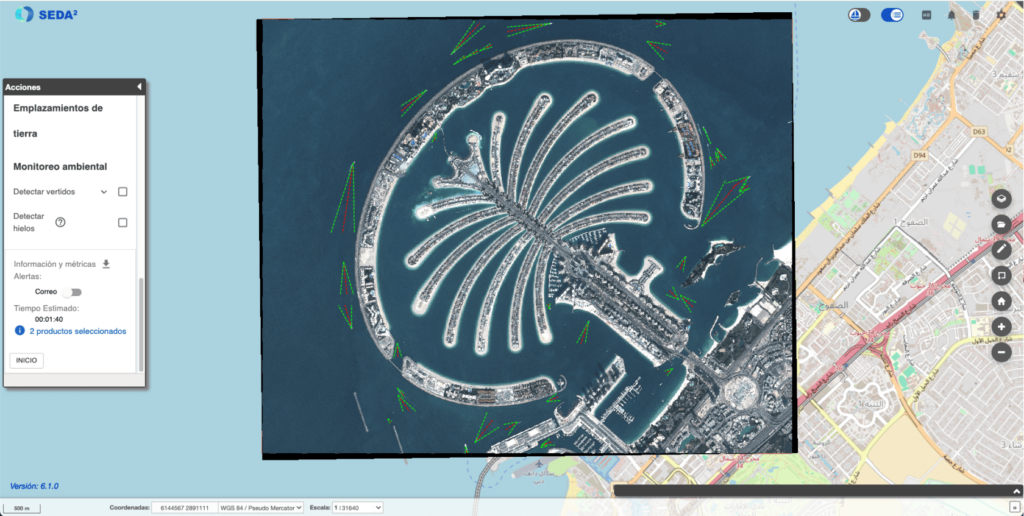
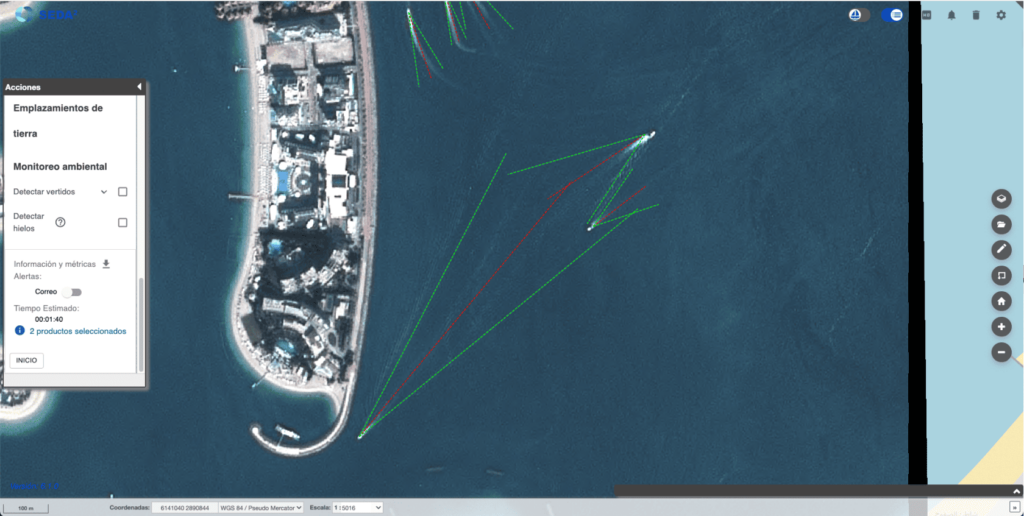
Figure 1 is a good example of how the wake of ships is much larger than the size of the ship, allowing in certain conditions to detect vessels that might not be identified with a detection model. Figure 2 shows in more detail some of these detections, where the model is able to identify the Kelvin wake and turbulent wake traces even when they overlap as they come from nearby ships.
As a result, SEDA offers the possibility to download reports in customized formats such as PowerPointor reports in standardized formats such as KML, NVG o ShapefileIn addition, reports in NATO formats such as ISRSREP y RECCEXREP format are also available NSIF.
Applications
The detection of both vessels and their wake provided by SEDA are particularly useful in maritime security and surveillance contexts, where a higher level of control over vessels seeking to remain hidden is allowed. Also in the fields of naval operations and rescue and salvage, where rapid detection of vessels in large search areas can be crucial to ensure the safety of the passengers.