Detecting and classifying ships in satellite images is crucial for multiple applications, from maritime security to commercial traffic management. It enables governments to monitor their territorial waters, combat illegal activities such as unauthorized fishing and smuggling, and protect marine ecosystems. It also facilitates the optimization of trade routes, the prevention of accidents, and the monitoring of pollutant spills. In emergency situations or natural disasters, it helps in locating ships in distress and in assessing damage.
In this scenario, SEDA addresses these challenges by using Artificial Intelligence techniques on satellite images of different electromagnetic spectra and/or resolutions. This approach manages to automate both detection and classification in an efficient and accurate manner.
Input and model characteristics
This use case supports different types of georeferenced images at the entrance: Images SAR-IW (Synthetic Aperture Radar – Adquisition mode Interferometric Wide, de 10m/px), and images Electro-optical (between ~1m/px and 10m/px resolution).
The Ship detection under SAR images, have a clear advantage over meteorological or lighting conditions, as they detect the energy emitted by the sensor itself and do not require sunlight, in addition to being able to penetrate clouds or unfavorable weather conditions. In contrast, a key challenge in ship detection is differentiating between ships and elements such as offshore platforms (Figure 1.b), which resemble ships in SAR images of 10m/px resolutions (Figure 1.a). To address these complications, SEDA uses advanced preprocessing and Convolutional Neural Networks (CNN) capable of analyzing the characteristics of the elements in the image, reducing false positives and improving accuracy.
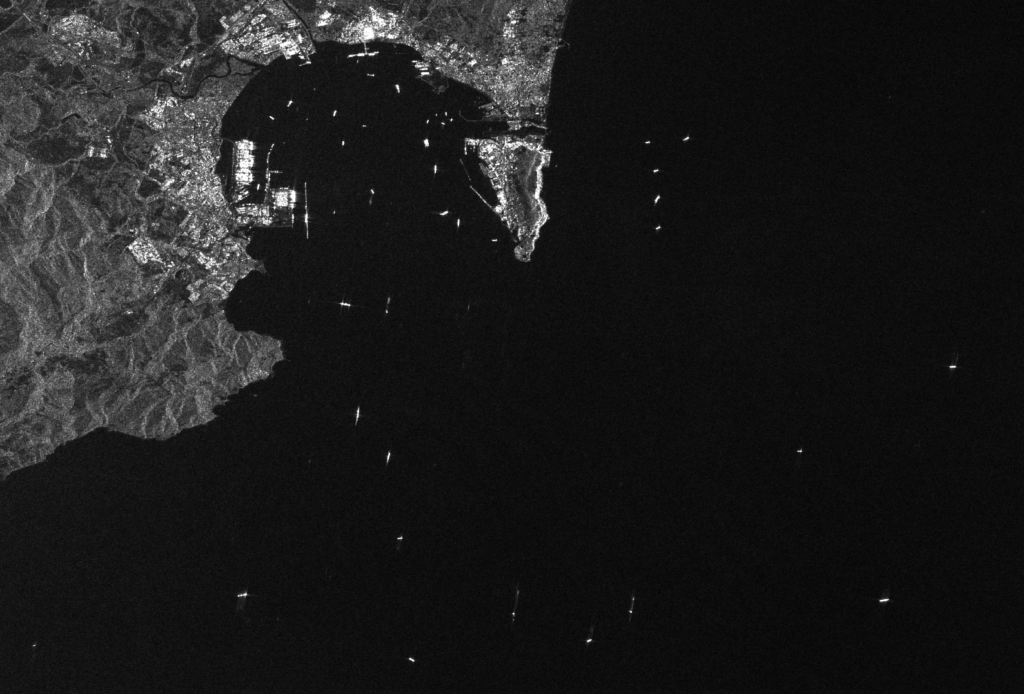
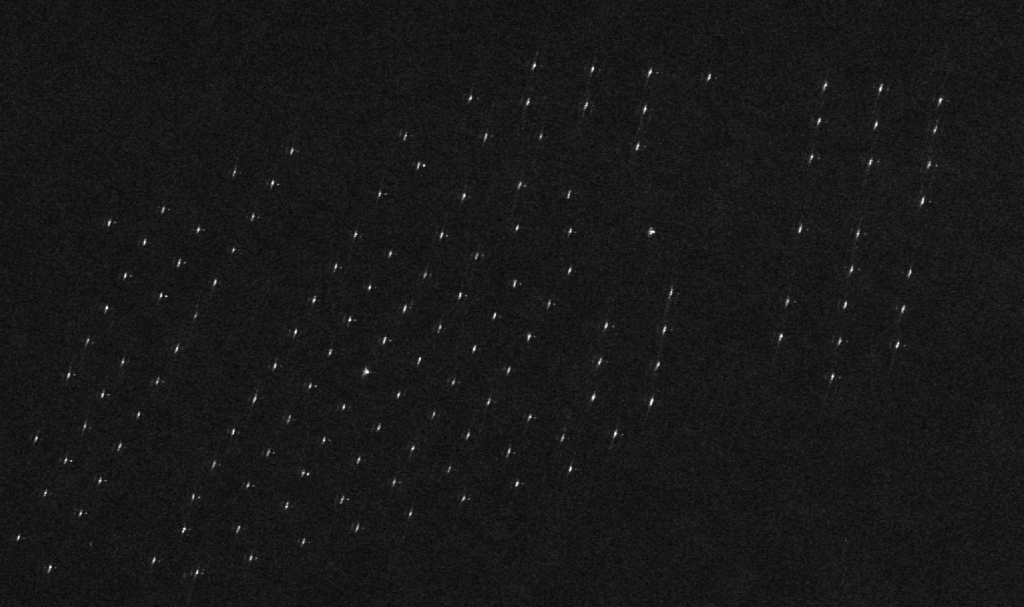
Another challenge focuses on the classification of ships in SAR images; the limitation of resolution is a factor to take into account, which is why use is made of CNN to extract characteristics such as an approximation of the length with which to classify the type of vessel. Likewise, there are other mechanisms used in SEDA, such as the use of AIS data that help detect and classify ships.
In contrast to SAR images, we have the optical images where adverse factors greatly affect the resolution of these use cases. This is why SEDA uses models based on CNN trained to solve different adverse conditions such as frozen seas, cloudy skies or low light. This adaptation allows the characteristics of the boats, such as shape, colours or size, to be extracted regardless of the adverse condition or the possibility of being partially covered or cropped.
In ship classification in 10m/px resolution optical images (such as Figure 2.), due to the low resolution, SEDA classifies with a civil/military level of granularity.
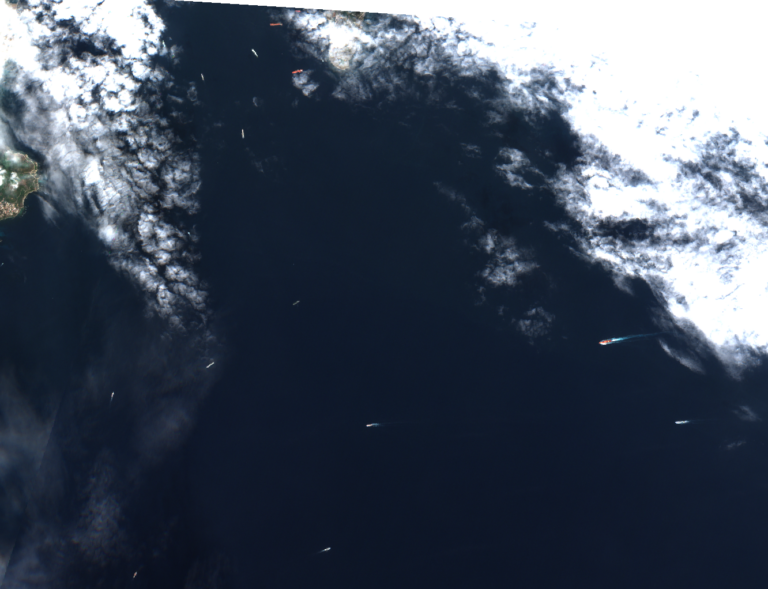
On the contrary, at resolutions less than or equal to 1 m/px (Figure 3.) the highest level of detail allows the classification in SEDA of up to 8 classes of vessels, focusing mainly on types of military vessels.
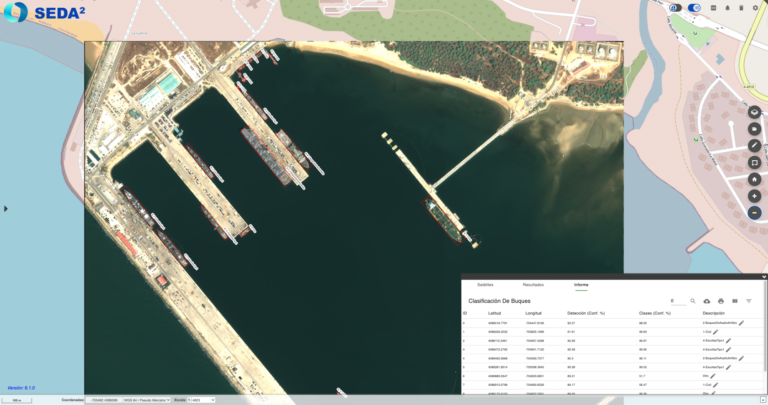
Output of the Artificial Intelligence model
SEDA automatically processes the output of the CNN neural network, displaying the results of the Overturned Box Detections (OBB) superimposed on a GIS viewer. Also, from SEDA the results are provided in formats such as shapefiles, KML y NVG; in addition to other types of official formats used in the NATO environment, such as ISRSPOTREP and RECCEXREP. The analyzed image converted to NSIF, a format designed for the exchange of images and related data in defense and intelligence environments. The versatility of these formats allows the Integration of SEDA results into other platforms and geospatial analysis systems, both civil and military.